Automatically creating digital twins of built environments
Digital twins have been starting to penetrate built environments because they can provide substantial benefits to all stakeholders. The process of creating such a digital twin is extremely time-consuming and requires very high human effort. This project aims to reduce the human effort in this process by proposing methods that apply advanced deep learning technologies to automate the process.
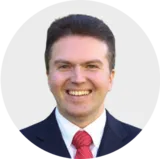
Focus Group: Built Environment Digital Twinning
Prof. Ioannis Brilakis (University of Cambridge), Alumnus Hans Fischer Senior Fellow
| Dr. Yuandong Pan (TUM, now University of Cambridge), Alumnus Doctoral Candidate | Host: Prof. André Borrmann (TUM)
Figure 1
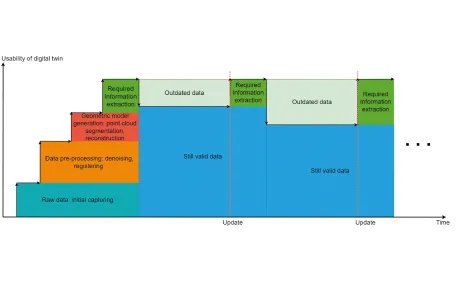
The adoption of digital twins (DTs) in the built environment sector is gradually increasing, as DTs can offer substantial value to all of the associated stakeholders. DTs can be used to construct, manage, maintain, and monitor physical facilities. There are mainly two reasons for this situation. The first reason is that many facilities have no pre-existing digital models from when they were constructed. Secondly, even if digital models exist, they are not updated throughout the assets’ life cycle. Hence, digital models are missing all asset modifications. This substantially reduces the reliability and usability of the data.
Data are continuously accumulated throughout the life cycle of the asset in the update process. However, it needs to be noted that more data cannot always guarantee a better representation of the facility. If we use the term “usability” to describe how accurate and useful the data in a digital twin will be, it needs to be noticed that although the data are continuously added to the digital twin throughout the life cycle of a facility, the usability of the digital twin would start to drop while the data are starting to be outdated. Subsequently, usability starts to be restored when the digital twin receives newly updated data. This concept is illustrated in Fig. 1.
Existing capturing technologies such as laser scanning or photogrammetry allow the automation of data acquisition for geometric models. However, the generation of semantically rich DTs is a complex process that is extremely labor-intensive. This project aims to reduce the human effort in this process by proposing methods that apply state-of-the-art deep learning technologies to automate the process.
The overall proposed approach, as illustrated in Fig. 2, uses laser-scanned point clouds and images as input. First, it starts with segmenting a point cloud of a multi-story building into individual stories. Subsequently, two different approaches are proposed for buildings depending on whether they do or do not fulfill the Manhattan-world assumption. Both approaches use the semantic information extracted by point cloud deep learning. The void-growing method, designed for Manhattan-world buildings, starts with extracting the void spaces inside rooms. Space-bounding elements are then extracted on the basis of the extracted void spaces. For buildings that do not fulfill the Manhattan-world assumption, planes in point clouds are extracted, intersected, and selected by a method based on energy optimization.
The next step is reconstructing small objects, where images are used to improve the results; this approach is based on the finding that recognition of small objects in images outperforms that in point clouds. The photos taken by the camera can be registered with laser-scanned point clouds by the photogrammetric process, which means the photogrammetric point cloud works as a bridge to fuse data from different sources. Subsequently, state-of-the-art artificial neural networks of image segmentation are implemented. Then, the recognized semantic information in the images is mapped to the laser-scanned point clouds by the camera matrices from the photogrammetric process. Predefined geometric primitives are then fitted to the point clusters with semantic information and added to the digital twin as simplified geometric information. In model enrichment, state-of-the-art deep learning models for text detection and recognition are applied to the images. Text information, such as serial numbers of objects and room numbers, is extracted from images and then mapped to the reconstructed objects in 3-D space.
The proposed approach in this project uses point cloud data and images as input to create an information-rich digital twin of indoor environments. The final output of the approach contains geometric and semantic information for space-bounding elements and small elements. The created digital twin can be used with regard to different aspects throughout various assets’ life cycles. For historical assets that have been completed for many years but do not yet have any digital records, the created digital twin can help to start and keep a recording of their current conditions for making better decisions, especially in facility maintenance and renovation. For those assets with available digital representations, the proposed approach provides the possibility to enrich the current digital model with more useful information as well. Keeping the digital twin dynamic and up-to-date can improve the asset’s real-time progress monitoring, quality control, diagnostics, and prognostics for facilities. Furthermore, the created final output can also be used in capital investment projects before the design and construction of the facility. It is efficient and convenient to simulate the performance with its digital twin to assist decision- and strategy-making in various predictive scenarios.
In practice, the proposed approach can heavily reduce the human effort in the process of reconstructing indoor environments from point clouds and photos. The human modelers do not need to measure the locations and dimensions of elements in the environments. Instead, they could just check the automatically created models and fix the corresponding parts if errors or inconsistencies are found. The cost to create a digital twin for indoor environments could be heavily reduced, which also provides the possibility to digitize more facilities. These digital twins can be used in various use cases and bring benefits to all stakeholders of the facility.
For the whole society, when more and more digital twins of various facilities are available, the current geometric conditions of these assets can be visually monitored. Furthermore, by putting different kinds of sensors into the physical assets and updating the corresponding data in digital twins, the data between digital and physical twins can be linked effectively. This could be a starting point to make smart buildings or smart cities. Decision-makers can effectively collect information for buildings and cities in the digital twin.
In the future, research could be conducted to address aspects such as the following: First, more component categories could be considered, such as staircases, handrails, furniture, and so on. The digital twin can be further enriched by including more object classes. Second, components with more complicated structures can be considered. Finally, data from other sensors can also be included, such as thermal sensors, which can enrich the information in digital twins. The multimodal data in digital twins can provide more potential use cases.
Selected publications
-
Brilakis, I. et al. Built environment digital twinning. International Workshop on Built Environment Digital Twinning presented by TUM Institute for Advanced Study and Siemens AG (2019).
-
Pan, Y., Braun, A., Brilakis, I. & Borrmann, A. Enriching geometric digital twins of buildings with small objects by fusing laser scanning and AI-based image recognition. Automation in Construction 140, 104375 (2022).
-
Pan, Y., Braun, A., Borrmann, A. & Brilakis, I. 3-D deep-learning-enhanced void-growing approach in creating geometric digital twins of buildings. Proceedings of the Institution of Civil Engineers-Smart Infrastructure and Construction 176 (1), 24-40 (2022).
-
Pan, Y., Braun, A., Brilakis, I. & Borrmann, A. Void-growing: A novel Scan-to-BIM method for Manhattan world buildings from point cloud. Proceedings of the 2021 European Conference on Computing in Construction (2021).
-
Pan, Y., Noichl, F., Braun, A., Borrmann, A. & Brilakis, I. Automatic creation and enrichment of 3-D models for pipe systems by co-registration of laser-scanned point clouds and photos. Proceedings of the 2022 European Conference on Computing in Construction (2022).