The value of monitoring for infrastructure management
Structural health monitoring (SHM) systems are potent tools for decision support. Our Focus Group’s research centered on quantifying the value generated through the systematic integration of SHM in the operation and maintenance decision-making process for engineering structures and infrastructure systems.
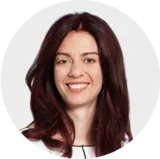

Focus Group: Data-Informed Decisions for Structures and Infrastructures
Prof. Eleni Chatzi (ETH Zurich), Alumna Hans Fischer Fellow (funded by the TÜV SÜD Foundation) | Antonios Kamariotis (TUM), Doctoral Candidate | Host: Prof. Daniel Straub (TUM)
(Image: Astrid Eckert (Chazi), Andreas Heddergott (Straub))
This report summarizes our Focus Group’s investigations into the application of structural health monitoring (SHM) systems for enhancing decision-making for engineering structures and infrastructure systems. Our research centered on evaluating and quantifying the impact of SHM on the operation and maintenance decision-making processes, highlighting its pivotal role in improving the management of engineering structures and infrastructure systems through data-informed decision-making.
In our first publication [1], we explored the concept of value of information (VoI) in structural health monitoring (SHM). Our focus was on understanding how long-term vibration-based SHM systems can assist in decision-making, particularly in maintenance planning. We introduced – for the first time in literature – a comprehensive framework that captures the entire process of SHM, from data collection to decision-making (see Fig. 1). This framework was applied to a bridge system example, demonstrating how to assess the optimality of SHM in different decision contexts.
Our second publication [2] presented a framework for quantifying the expected gains that continuous SHM-aided maintenance planning can provide when compared against the currently dominant approach of intermittent inspection-based maintenance planning. A novel metric, the value of SHM (VoSHM) metric, was introduced for formally computing these expected gains. The presented framework can be used as an actionable decision support tool for guiding decisions on whether or not to install an SHM system on a structure, for a wide range of SHM use cases. Using a bridge model, we explored several actionable SHM use cases, demonstrating the substantial economic advantages of SHM in certain decision-making contexts.
Stochastic models describing time-evolving deterioration processes are instrumental for facilitating a predictive maintenance planning paradigm. In the modern data-centric landscape, Bayesian methods can exploit monitoring data to sequentially update knowledge on underlying model parameters. The precise probabilistic characterization of these parameters is indispensable for several real-world tasks, where decisions need to be taken in view of the evaluated margins of risk and uncertainty. Our third publication [3] investigated and compared on-line and off-line Bayesian filters and adapted the former for posterior uncertainty quantification of time-invariant parameters of deterioration models. We showed that tailored on-line particle filters are competitive alternatives to computationally prohibitive off-line Bayesian filters.
In our fourth publication [4], we focused on purely data-driven prognostic algorithms based on monitoring. Specifically, we proposed a novel metric for assessing and optimizing such algorithms in the context of predictive maintenance (PdM). This metric, distinct in its approach, uses the algorithms’ output – predictions of the system’s remaining useful life (RUL) – as inputs to PdM decision-making policies that eventually inform decisions. The application of the proposed framework for evaluating this metric, shown in Fig. 2, was demonstrated using theoretical and real-world case studies, including one involving degrading turbofan engines.
In addition to the above publication output, our Focus Group engaged with global leaders in related research areas, organizing a workshop and an online speaker series. The virtual workshop in October 2020, titled Frontiers in Monitoring-Supported DecisionMaking for Structures and Infrastructures, included presentations from ten research leaders.
Its primary aim was to foster extensive discussions and idea exchange. Following this, in May and June 2021, we held a speaker series featuring 12 experts, which attracted a wide audience, including TUM’s Master’s students. This collaborative knowledge exchange led to drafting a position paper on Monitoring-Supported Value Generation for Managing Structures and Infrastructure Systems [5] (see Fig. 3), submitted in January 2024.
Overall, this project contributed to the deepening of our understanding on the means and ways by which SHM impacts the decision-making process. We believe that interdisciplinary, collaborative efforts, similar to the one conducted in our Focus Group, are key to reaching a reliable synergy between SHM and decision-making.
Figure 2
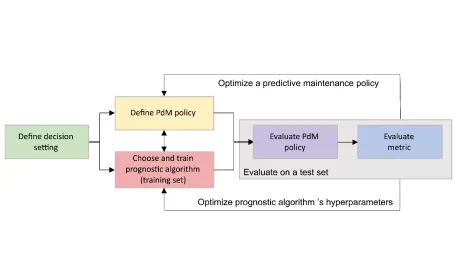
Figure 3
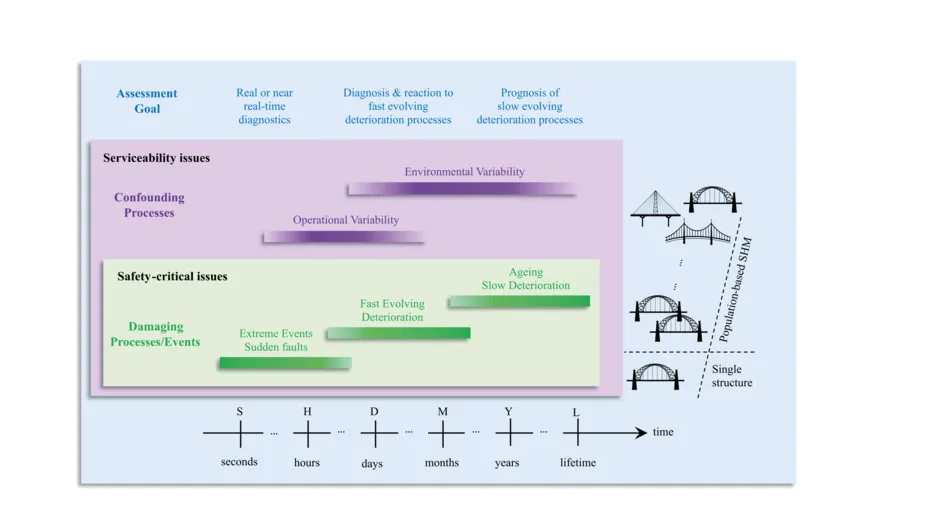
[1]
Kamariotis, A., Chatzi, E. & Straub, D. (2022).
[2]
Kamariotis, A., Chatzi, E. & Straub, D. (2023).
[3]
Kamariotis, A., Sardi, L., Papaioannou, I., Chatzi, E. & Straub, D. (2023).
[4]
Kamariotis, A., Tatsis, K., Chatzi, E., Goebel, K. & Straub, D. (2024).
[5]
Kamariotis, A. et al. (submitted 2024).
Selected publications
-
Kamariotis, A., Chatzi, E. & Straub, D. Value of information from vibration-based structural health monitoring extracted via Bayesian model updating. Mechanical Systems and Signal Processing 166, 108465 (2022).
www.doi.org/10.1016/j.ymssp.2021.108465. -
Kamariotis, A., Chatzi, E. & Straub, D. A framework for quantifying the value of vibration-based structural health monitoring. Mechanical Systems and Signal Processing 184, 109708 (2023). www.doi.org/10.1016/j.ymssp.2022.109708.
-
Kamariotis, A., Sardi, L., Papaioannou, I., Chatzi, E. & Straub, D. On off-line and on-line Bayesian filtering for uncertainty quantification of structural deterioration. Data-Centric Engineering 4, e17 (2023). www.doi.org/10.1017/dce.2023.13.
-
Kamariotis, A., Tatsis, K., Chatzi, E., Goebel, K. & Straub, D. A metric for assessing and optimizing data-driven
prognostic algorithms for predictive maintenance. Reliability Engineering & System Safety 242, 109723 (2024). www.doi.org/10.1016/j.ress.2023.109723. -
Kamariotis, A., Chatzi, E., Straub, D., Dervilis, N., Goebel, K., Hughes, A.J., Lombaert, G., Papadimitriou, C., Papakonstantinou, K.G., Pozzi, M., Todd, M. & Worden, K. Monitoring-Supported Value Generation for Managing Structures and Infrastructure Systems. Submitted to: Data-Centric Engineering (2024).