In Focus:
From Urban Air to Global Climate
Atmospheric chemists at Harvard, led by Frank Keutsch, probe basic processes taking place in the air. Jia Chen’s group at TUM charts emissions of greenhouse gases and other pollutants with high resolution. Together they are generating new knowledge relevant to both human health and climate change.
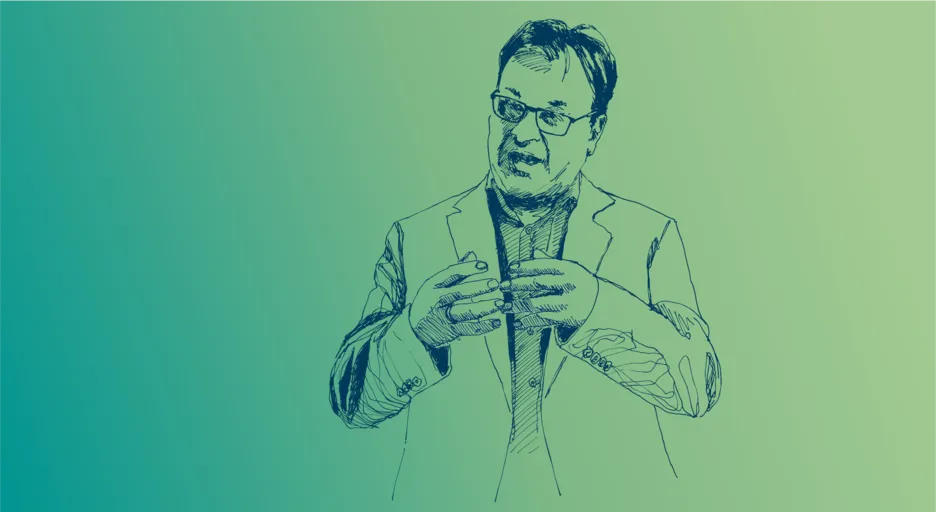
The air we breathe, the climate we bequeath
Except in smoggy cities, the air we breathe is normally invisible. Yet it’s plain to see that we depend on it, we interact with it, and we have changed it in ways that can harm human health, upset ecological balance, and even threaten civilization. For decades, the saying “out of sight, out of mind” has gone a long way toward explaining why many environmental problems of the Anthropocene – not just air pollution and global warming, but also phenomena such as the “sixth mass extinction” that appears to be under way – remain poorly understood and ineffectively addressed. Here science and engineering have a role to play, a challenge taken up by the TUM-IAS Focus Group on Air Pollution and Climate.
Based at Harvard University, Hans Fischer Senior Fellow Frank Keutsch is a professor of Engineering and Atmospheric Science as well as Chemistry and Chemical Biology. His Host, Jia Chen, is a professor of Environmental Sensing and Modeling in the Department of Electrical Engineering at TUM. They are uniting largely complementary expertise in this Focus Group, with the ultimate goal of enabling policy makers to assess potential approaches to improving air quality and combating climate change.
For a closer look at how this collaboration works, what the researchers have learned, and what they envision for the future, science journalist Patrick Regan spoke with Frank Keutsch and Jia Chen by videoconference. Their conversation has been edited for clarity and length.
Q: Your collaborative research ranges from basic chemistry to engineering and on to implications for public policy. What are you trying to find out? Why? And how?
Keutsch: There are two reasons that come together why I and, I believe, Professor Chen, are conducting the research that we are doing. One is just trying to understand the world around us, in reality a small aspect of the world around us, and get an in-depth mechanistic understanding of how different aspects and processes couple together, and how they form the world around us. In our case that has to do with the atmosphere and air quality, and then both Professor Chen and I do work in the direction of climate more broadly. It’s really a question of how well we understand the air that we breathe, its composition, and what changes it. Part of that, at least for me, is a purely intellectual curiosity. I want to understand things. I find it frustrating when there’s something I find fascinating that I don’t understand.
Then again, of course, this is in many ways applied research, so there’s also the motivation for the application. And that, fundamentally, is that if we better understand the air that we breathe and that interacts with plants and other things, perhaps we can also find ways to improve that air quality so that it has less harmful side-effects. In a sense, it is an applied science, so it is driven by the applied program of studying and improving air quality.
Chen: In my team, we are really good at developing sensors, and sensor networks, as well as automation and communications. And Frank’s group is very strong in atmospheric chemistry. We are developing sensor networks and gathering the data, and together with Frank we can understand better how the metabolism of air pollution in the cities works, what are the drivers for the air pollution, where the air pollution is coming from, and so on.
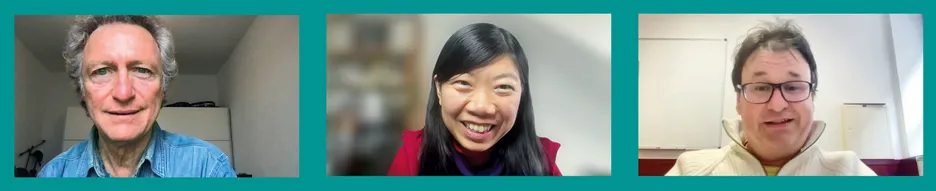
Q: You’re dealing with quite a range of time scales, aren’t you?
Keutsch: The reason things are greenhouse gases is typically that they live a long time in the atmosphere, whereas the reactive species, usually from air pollution, are pretty short-lived. And so one of the big distinctions, for example between the greenhouse gases CO2 and methane, is that CO2 really does not get chemically processed in the atmosphere. It’s in there, and it gets taken up by the earth’s surface in some form or another. Methane actually can be chemically processed in the atmosphere, and then it’s turned into CO2, a less effective greenhouse gas, but nonetheless, a greenhouse gas. And this turnover rate of methane into CO2 is determined primarily by one of the highly reactive species, the OH radical. It’s a species that is very transient but is really the key to determining the atmospheric lifetime of methane.
You have to know the emission sources and the chemistry, and then you also need to know the different things that can happen, for example, when it gets deposited in the ground or taken up by plants. Uncertainty in any of those will drive uncertainty in what you predict a certain concentration will be.
It’s like a three-legged stool. You need a combination of measurements in the real world, models that try to represent the real world, and detailed specific studies of reactions to try to improve their representation in the models.
Chen: Also, in the context of urban air monitoring, we have been able to detect and analyze changes over a period of days or weeks. What we learn could make it easier to predict how conditions might change on a comparable time scale.
Keutsch: There is also the question of how much humans have actually changed the world around us, in this case the air that interacts with living things, and to what degree we can control that. What’s really interesting is the question: What is this interaction between things that we generally think of as natural and the human part? In some cases those can in essence build on each other and result in much worse air pollution, and in other cases they don’t. What role do humans play, at a mechanistic level? One of the things we often want to know is how we’ve shaped and formed this planet. How well do we actually know what the planet was like before human interaction? People always talk about pre-industrial conditions. For many things, we can go back to pre-industrial conditions. You can take an ice core and see how much CO2 is in it. We can go back and look at all kinds of historical records.
But when it comes to the chemical drivers of air pollution, there’s an interesting conundrum: The actual compounds that drive chemical transformations in the atmosphere will be, by definition, very reactive. A chemical process will follow the easiest path, the fastest path, and that’s the one that matters. That means these compounds do not survive in ice cores. To represent these correctly in models that can say, this is the condition pre-industrially, we have to rely on a fundamental understanding of chemical processes involving these most reactive species, commonly called radicals
“The ability to look back can give us confidence, not just for climate models, but also for reactive compounds that make air pollution.”
Frank Keutsch
Q: Does that involve volatile organic compounds?
Keutsch: Those are the things that get transformed by radicals. Volatile organic compounds are also reactive and mostly wouldn’t survive in an ice core. There’s a whole range of reactivity. The only way we can travel back in time and have an accurate understanding of the processes that would occur, and how they would occur, is if we understand the actual chemical processes.
Q: So you can use proxies and models to work out what’s happening?
Keutsch: Exactly. So then we can say, we know these reactions, we know how these behave, and then you can integrate them into a model. For those things, the only way to travel back in time is then in “model world.”
Q: What are some examples of these highly reactive substances?
Keutsch: Nitrogen dioxide or NO2 is a radical. This is a part of NOx that we’re concerned about when it comes to cars and combustion. There’s the OH radical. Even ozone, which is not a radical but is highly reactive, can’t survive well in long historical samples. In a way, we use models not only to travel back in time, but also when we want to predict the future. The ability to look back can give us confidence, not just for climate models, but also for reactive compounds that make air pollution. That also gives us some confidence that we understand the processes that would shape a future atmosphere where we’ve changed emissions into the atmosphere.
In my group, we have primarily specialized on measurements of an intermediate reactivity range. As hydrocarbons get emitted, those typically get processed via oxidative processes. We look at the precursors, the hydrocarbons, as well as the reaction products that are formed, and how fast this processing happens, from emissions into pollutants – in part to see where we need to have additional understanding.
We use tools from spectroscopy, an interaction of light with molecules and particles. We also use mass spectrometry, where we try to use the mass of individual molecules to identify them. Professor Chen’s group uses primarily spectroscopy, but also electrochemical sensors, to measure some of these compounds we’re interested in.
My group does this across a wide range of conditions. We try to find places on the planet that have very different conditions, that perhaps in some respect can get us closer to pre-industrial. We’ve done measurements in the middle of the Amazon, where there’s very little of certain types of human interactions. That should be, in some coordinates, still fairly close to pre-industrial. We also do measurements in urban areas that are clearly anthropogenically influenced
Q: Do you have data from polar regions?
Keutsch: Yes, we’ve done measurements on the ground and on ships. We were on an icebreaker going to Antarctica. Recently we’ve been doing a lot of measurements on stratospheric aircraft that go high into the atmosphere. We just did some measurements from Alaska. We’ve done that over the United States in the past few years with the idea of trying to understand these chemical processes under as many different conditions, in as many different places, as possible. Because after all, if we understand the processes correctly, we should be able to understand them regardless of where we are looking.
Now and again you find surprises. You may see a process you hadn’t thought of before that you suddenly realize exists. And then you do laboratory studies. The problem is that the atmosphere is a messy place, especially the troposphere where we live. When we find something surprising, we typically go back to the lab to study that process in isolation, without all the uncertainties that the real system introduces. In my group, we do field measurements, and then lab measurements that tell you the detailed processes including reaction rates, how fast they occur. And then we go to models and see whether integrating that into the models actually improves agreement between the virtual model world and observations in the real world.
It’s like a three-legged stool. You need a combination of measurements in the real world, models that try to represent the real world, and detailed specific studies of reactions to try to improve their representation in the models.
Q: Looking at the present state of the atmosphere with an eye toward gaining a better understanding of both air pollution and climate change, you also have relatively high-resolution data from what could be called a “mission to Munich.” Could you give an overview of that work?
Chen: MUCCnet, the Munich Urban Carbon Column network, is a worlwide unique city sensor network measuring the total air column concentrations of greenhouse gases using the sun as a light source. We have sensor stations located both within and outside of Munich. You can look at the difference between the concentration in the column measured before and after the city, and the difference is a measure of the emission of the city.
Because of this uniqueness and also my expertise in developing sensors and models, Munich has been selected as a pilot city in the ICOS Cities PAUL project within the European Green Deal. ICOS stands for Integrated Carbon Observation System, and PAUL stands for Pilot Application in Urban Landscapes. The aim is to develop novel methods for monitoring city emissions, and they chose three pilot cities: Munich, Paris, and Zurich. I am the scientific lead for Munich. Within this project, we are adding more monitoring capacity on top of MUCCnet. We have 20 roof-level sensors, 100 street-level sensors, and then air quality sensors. So we have more than 170 sensors right now in Munich, to observe both greenhouse gases and air quality.
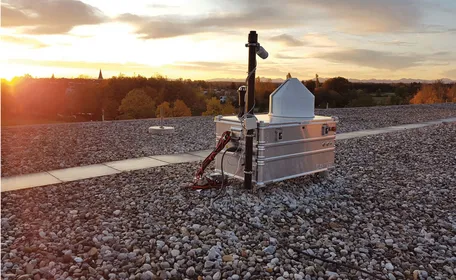
Q: Particulate matter as well as gases?
Chen: Yes, though only some of the sensors detect particulates. It’s an integrated sensor network that is measuring all kinds of species that are relevant for climate and health.
Q: How does that compare to the other cities involved in this EU project?
Chen: Each city has its own strength. MUCCnet is the strength of Munich. Some aspects are the same for all three cities. For example, Munich has roof-level sensors, same as Zurich and Paris. But only Munich and Zurich have street-level sensors, and only Munich and Paris conduct total column measurements.
Q: Given that some of the sensors rely on sunlight, what do you measure at night?
Chen: Well, it is difficult to measure anything at night if you use sunlight. Many people are saying that satellite observation is the future. If so, we can also use MUCCnet to validate satellite measurements, to make the satellite data more accurate and then benefit from the global coverage. That’s its advantage. But we also have sensors operating all the time, using an infrared light source inside the sensor. The disadvantage is that these are surface sensors measuring concentrations just at one point. The column sensor provides more information because it uses the sun as the light source and then integrates the concentration along the air column and correspondingly provides two-dimensional information instead of just one point.
Q: What do you do to get the most information and knowledge out of the data?
Chen: We are developing a modeling method, based on mathematical principles and employing machine learning, to localize, quantify, and characterize the emissions. This is also part of my successful proposal for the ERC Consolidator Grant, which is funded by the European Research Council.
Q: Let’s talk about how the models work and how they’re used. Do you come to the models with hypotheses and test them in the model, and then test the model against the data?
Chen: It can be thought of as two steps. You want to do some scientific experiment. You say you know the truth, and you test your model and see how well your model can recover the truth. And if you know that, and we have quantified the model’s capability, we can deploy the model to the real-world data to get the emissions. In addition, you can imagine that we are measuring concentrations at different locations, in Munich for example, and we want to know where the emissions are coming from, how much a power plant or a car emits. Some sort of translation needs to be done between the emissions and the concentration that is measured. So this is what the model is supposed to be doing. That requires some knowledge of atmospheric transport. It requires mathematical knowledge as well. So together we can build the linkage, or what we refer to as the transfer function. Machine learning can help with this.
Q: What are the benefits and limitations of machine learning in terms of extracting knowledge from data and applying that knowledge to real-world conditions?
Chen: The model is partly to build this transfer function between the concentrations and the emissions. But machine learning can also help in making the sensor values more accurate. That’s one application. And then secondly, if we measure concentrations, it’s not the whole picture. Machine learning can also help us to interpolate the whole concentration field from the measured, sampled points to the whole map, for tracking air pollution in cities for example.
Machine learning can also help us to gain finer resolution for satellite pixels. Let’s say you want to understand the urban biosphere, how much carbon is taken up by Westpark or the English Garden. You have some satellite observations. Still, that is very difficult to determine the urban biospheric fluxes. While the vegetation is doing photosynthesis, a certain amount of light is emitted back to space, usually in the red or infrared range. The satellite detects that part, and this is an indication of the photosynthetic activity of the vegetation. However, the satellite measurement pixel is very very coarse, say, five kilometers. You can have only a few pixels in Munich, and certainly you cannot determine the photosynthetic capacity of Westpark or the English Garden. Therefore, you need to develop some super-resolution technique, and that is what we have done using machine learning.
Keutsch: Machine learning can be very efficient computationally. And as an exploratory tool, I think it’s unbeatable, because it’s much cheaper and faster.
We are both working to integrate machine learning and AI into our toolkits. Professor Chen’s group has more expertise than my group in tools that have to do with detailed fluid dynamics modeling, for example air flow in the complex environment of a city. Where my group has more expertise is in the details of the chemical mechanisms and transformations. We do modeling as well, but using what’s called chemical box models or chemical transport models.
Now, the way you have to imagine a chemical transport model is that it is trying to account for all the processes it can that exist in the real atmosphere, for every individual location. Each location has a certain size, and if you want to go to a more regional scale, you need a higher resolution. This increases the computational effort. Also, you’re trying to include emissions into that, and a myriad of chemical reactions, transport processes, turbulence, and more. That is computationally quite expensive. In a way, you could say they’re so computationally expensive that we can’t possibly include all the processes that each research group thinks it’s important to do. If you find a new chemical reaction, you really need to convince the chemical modelers that it’s worthwhile for them to add another reaction into their model.
Machine learning models, on the other hand, are quite ignorant of all this. They have a lot of data available, which you can use to train them, and in a sense they’ll find their own way to represent that internally. Which intellectually – I’ll say as an atmospheric chemist – is incredibly frustrating. Because it says: Who cares about this great detailed chemical reaction? About two years ago we found an absolutely fascinating phenomenon where formaldehyde can be a catalyst for the formation of sulphate particles, really weird.
Modeling with machine learning might make it easier to have decentralized, short-term forecasting of air quality. It’s now being used for predicting weather, and if you stay in the short-term range, machine learning models are currently competitive with the big complicated models. If you try to predict farther out, they start going weird. This is not a replacement. My chemical transport models, like climate models, can run quite a bit into the future. But machine learning might potentially enable short-term air quality forecasting, perhaps even tailored to a specific region where you don’t have measurement stations but want to make predictions.
We used it to predict changes in ozone, for example. It did a really good job of predicting this if you fed it with the right information. The idea is that you don’t let it run free, but you set some of the boundary conditions, and this gets updated. It did quite well. The reason that matters is that we have air quality measurement stations only in very few locations. It’s expensive, it takes effort, and they are predominantly in urban areas.
Q: So when a machine learning approach yields results that are helpful in understanding real-world conditions, can it be transferable to different situations?
Keutsch: Possibly, within limits. Transferability will likely be limited to locations that span the same parameter range as where you trained your machine learning model.
Q: Could it be useful in assessing the effectiveness of mitigation efforts?
Keutsch: Yes, to the degree your mitigation efforts don’t start moving you outside of the training data or boundary conditions that were available.
Q: One striking result from the Focus Group is your study of the air in and around Munich before, during, and after Germany’s Covid lockdown period. Was this to some extent a proof of concept for your measurement, modeling, machine learning, and analytical methods? And what did you learn?
Chen: That was a very nice collaboration, which shows that together we can have really nice results. This work was done by my PhD candidate Vignesh Balamurugan, together with Frank and myself. Covid was very unfortunate, of course, but at the same time you see the emission reduction and a chance to study what kind of impact such a reduction in emissions has in terms of air pollution levels in cities.
Keutsch: If we went to the mayor’s office in Munich and said, could you please stop all traffic for some number of days to reduce NOx, because we want to see whether our models and our understanding are right, we would get laughed out of the place. But Covid did it for us. Especially during the harder lockdown, traffic was reduced dramatically.
Chen: This enabled us to study what happened to several kinds of pollution. For most people, if you talk about air pollution, especially in German cities, they think about NOx. That’s what people are talking about in the media, often in relation to diesel bans or the diesel scandal. NOx is very relevant to health. People don’t think too much about ozone and particulate matter, but these too are very harmful air pollutants, very harmful for health.
Keutsch: Ozone is a gas, fairly reactive and fairly short-lived, and particulate matter is much longer-lived. Both are of large significance, as is NO2 itself, or NOx, which is a mixture of NO2 and NO. Put simply, the more hydrocarbon emissions you have, the more pollution you’re going to get. More ozone, more particulate matter. But how much you actually get depends on NOx, and it has a complicated dependence. The only source of ozone in the troposphere is actually photolysis of NO2. The first thought, of course, is the more NO2 I have, the more ozone I’m going to make. However, through some very complicated coupled chemical reactions, what happens is at some point you have so much NOx that it actually suppresses the chemistry of ozone formation in the end, and with a further increase in NOx you actually have less ozone pollution.
Chen: What happened in the Covid lockdown is that we saw the NOx emissions reduced a lot, which was expected because we had less travel, less car transportation. But then ozone actually increased, and the particulate matter concentration stayed constant. So it’s not a very simple story. OK, we reduced NOx, but that doesn’t solve all our pollution problems. In this case ozone increases, because in German cities, the air is actually in a NOx-saturated regime. That requires atmospheric chemistry to understand what’s happening. In a NOx-saturated regime, if you reduce NOx, then ozone increases. So you need to reduce NOx much more, and then we can go to the other regime, which is a NOx-limited regime, so that ozone can also decrease.
Keutsch: Indeed, in all the larger urban areas in Germany, as Covid reduced NOx emissions, ozone went up compared to the previous year. We had to do a lot of checking, but finally, I would argue, it proved that we understand the general chemistry of it, and that we also understand the current status of German cities.
Chen: It is very important to understand which regime we are currently at in the German cities, and it turns out that we are in the NOx-saturated regime. Therefore, in terms of environmental policy, we need to consider this as well as other factors.
Keutsch: As we transition, let’s say more and more to electric cars, and NOx goes down, that means ozone will initially increase until we get over that bump. I want to be very careful that things don’t get misrepresented. No one should be able to say NOx emissions are good because they reduce ozone. The reason they do that is because we have such high NOx levels at present.
Chen: Either we reduce the NOx enough to reach the goal of ozone reduction also, or we need to really understand the atmospheric chemistry and think about the tradeoff.
Q: Did you gain any new insights into particulates?
Keutsch: Particulate matter is a much more complicated process. Lots of things contribute. Particulate matter you see in location A, as it lives longer, is influenced much more by being produced in some upwind location. And what we found is that while the reduction in NOx had a clear impact on the ozone, the particulate matter concentrations remained unchanged. We can’t model particulate matter to the same degree of trust, I would say, as we can model ozone.
What we saw in our study is that reductions in NOx left particulate matter virtually unchanged. We were discussing what this might mean, and I had my own very convinced hypothesis, and Vignesh countered that it was more complicated and might have to do with the combination of nighttime and daytime chemistry. He did some further research and showed me he was right. There are many contributors to aerosols, and daytime production is associated with different chemical processes than nighttime. The idea is that it was buffered in that respect, by shifting away from one type of aerosol and more to another type, so the net amount stayed roughly the same. There’s still a lot of work that needs to be done to really understand particulates better.
Q: What other recent or upcoming research efforts would you like to highlight?
Keutsch: We are joining together to do some actual atmospheric measurement campaigns in Munich. The first of these took place in August 2023. My instruments come from the US in a container. The instruments of the Chen group are already there, of course. We also have instruments coming from KIT in Karlsruhe, and perhaps we’ll also get one from China. The aim is to make very detailed measurements of the chemistry of the formation of ozone and particulate matter. We’re doing very intensive campaigns to measure as much as we possibly can to try to understand the formation of aerosols. We were delayed significantly by Covid and trickle-down effects, but there will be quite a lot more output from this TUM-IAS effort over the next few years, where we look at measurements and modeling.
Q: Do you see your collaboration continuing further, and deepening or broadening to involve other groups with different expertise and interests?
Keutsch: Once we have our findings and we understand the foundations of what’s happening, with regard to air quality in particular, I would be very interested in broadening the collaboration. At that point we will have a stronger basis for cooperation with people who understand public health, with people who understand the policy aspects, like Miranda Schreurs, or with the city of Munich.
With regard to climate change, I would also be very curious about broadening into
the issues surrounding climate intervention. The Chen group is very focused on greenhouse gases, which are the cause. My group, while working on a general understanding of stratospheric science, is also looking into the feasibility and potential side-effects of measures like stratospheric aerosol injection. These are quite complementary fields. One is trying to tackle the cause, and the other one is thinking about a potential Plan B if we do not address climate change rapidly enough. Some argue that no one should be doing research on this at all, because it’s not a long-term solution. My concern is that if there is serious discussion of intervening in natural processes on such a scale – and there is – someone other than vested interests should be studying the matter scientifically and thinking seriously about what approval processes would be appropriate.
Q: Returning to your goal of equipping decision makers to deal with air pollution and climate change, what can you do as scientists? How can you make effective connections between research and policy?
“What we can do is provide scientific evidence and facts to the politicians, and we hope that they can make wise decisions based on our findings to improve climate and reduce air pollution.”
Jia Chen
Keutsch: In my view, the foundation of policy really should be on the physical sciences. What we as physical scientists do is provide information that then can be used by economists, and social scientists, and political scientists, to think about decision making. Especially when it comes to climate issues, there’s a tendency to ask physical scientists about what decisions ought to be made, and I’m always apprehensive about that. Because I don’t have any training in that, I have no mandate, and the actual decisions have to involve not only economics but also public perception and what the public wants. One of the key things is to have effective interaction and intermediaries between the physical science world and the world of economics, social science, and political science. That is one of the challenges, where you need to have very specialized people who in a sense understand both worlds, if you want to do it effectively.
Chen: What we can do is provide scientific evidence and facts to the politicians, and we hope that they can make wise decisions based on our findings to improve climate and reduce air pollution. I’m not developing policies or making decisions. But I hope that these kinds of scientific findings, such as the example of the Covid lockdown investigation, could bring more clarity to the politicians. We show measured emissions and concentrations to the politicians so they can, for example, have a better overview picture. This includes providing information on previously unknown emission sources. When decisions are to be made about where to make reductions, this may help them define a priority source to reduce first.
Over the last eight years, I’ve tried to make connections with people in the city of Munich. And now I have good connections at the city level in a number of different departments. I would say that’s a success. I also got the city to support participation in the ICOS Cities project. This is very good, that they see the necessity of measuring greenhouse gases and not just calculating them, and that they support this project in particular. Within the project they are also providing us with a lot of information that will help us accomplish the science.
We organize workshops, inviting city officials and other stakeholders to attend. Here I’m working with TUM Professor Miranda Schreurs, the chair of Climate and Environmental Policy at the Bavarian School of Public Policy. [See “Pollutants and Sustainability Governance” in the TUM-IAS Annual Report for 2020.]
TUM President Thomas Hofmann and I hosted a rooftop visit to MUCCnet by Bavarian Minister President Söder, who was very interested in our work. He commented on our sensor systems as “Small box, big impact” (German: kleine Kiste, große Wirkung).
I’d say all of this shows we’re making headway, and while our main focus is on the research itself, we will keep pushing to make sure the results are available to people who can make a difference in addressing air pollution and climate change.
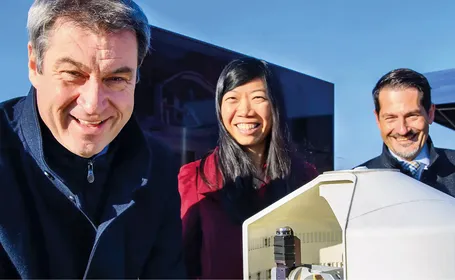