Chenchen Liu
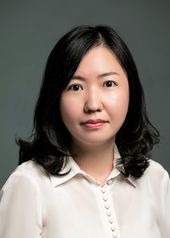
Fellowship
Hans Fischer Fellowship
Appointment
2023
Institution
University of Maryland, Baltimore County
Department
Computer Science and Electrical Engineering
Focus Group
Enabling Neuromorphic Computing for Multi-Tenant AI
Short CV
Dr. Chenchen Liu got her Ph.D. degree from Electrical and Computer Engineering Department of the University of Pittsburgh under supervision of Dr. Hai (Helen) Li in 2017. She is now an Assistant Professor of Computer Science and Electrical Engineering Department at University of Maryland, Baltimore County. Her works have been tremendously published in top-tier conference and journal. Dr. Liu has served as TPC Chair and TPC Track Chair for conferences DAC, GLVLSI, Cloud Summit, and etc. Dr. Liu is a member of IEEE CASS NG-TC. She also serves as an Associate Editor for Journals of TCAS-1 and Neurocomputing.
Selected Awards
- 2023, NSF Career Award
- 2022, Best Poster Award, The 5th Conference on Machine Learning and Systems (MLSys)
- 2014, Best Paper Award, IEEE Computer Society Annual Symposium on VLSI (ISVLSI)
Research Interests
- Novel computing systems and architecture for artificial intelligence
- Brain-inspired computing
- Non-volatile memory
- Hardware and software co-design
- Customized circuit and chip design
Selected Publications
Please see a full list of publications here: https://scholar.google.com/citations?user=sCLaaF0AAAAJ
- F. Yu, S. Bray, D. Wang, L. Shangguan, X. Tang, C. Liu, and X. Chen, "Automated Runtime-Aware Scheduling for Multi-Tenant DNN Inference on GPU," 2021 IEEE/ACM International Conference On Computer Aided Design (ICCAD), Munich, Germany, 2021, pp. 1-9, doi: 10.1109/ICCAD51958.2021.9643501.
- C. Liu, F. Yu, Z. Qin, and X. Chen, "Enabling High-Efficient ReRAM-Based CNN Training Via Exploiting Crossbar-Level Insignificant Writing Elimination," in IEEE Transactions on Computers, vol. 72, no. 11, pp. 3218-3230, Nov. 2023, doi: 10.1109/TC.2023.3288763.
- F. Yu, Z. Xu, C. Liu, D. Stamoulis, D. Wang, Y. Wang, and X. Chen, "AntiDoteX: Attention-Based Dynamic Optimization for Neural Network Runtime Efficiency," in IEEE Transactions on Computer-Aided Design of Integrated Circuits and Systems, vol. 41, no. 11, pp. 4694-4707, Nov. 2022, doi: 10.1109/TCAD.2022.3144616.
- B. Li, B. Yan, C. Liu, and H. Li, “Build Reliable and Efficient Neuromorphic Design with Memristor Technology,” In Proceedings of the 24th Asia and South Pacific Design Automation Conference (ASPDAC '19). Association for Computing Machinery, 224–229. https://doi.org/10.1145/3287624.3288744
- C. Liu, Q. Dong, F. Yu and X. Chen, "ReRise: An Adversarial Example Restoration System for Neuromorphic Computing Security," 2018 IEEE Computer Society Annual Symposium on VLSI (ISVLSI), Hong Kong, China, 2018, pp. 470-475, doi: 10.1109/ISVLSI.2018.00091.
- F. Liu and C. Liu, "Towards Accurate and High-Speed Spiking Neuromorphic Systems with Data Quantization-Aware Deep Networks," 2018 55th ACM/ESDA/IEEE Design Automation Conference (DAC), San Francisco, CA, USA, 2018, pp. 1-6, doi: 10.1109/DAC.2018.8465849.
- C. Liu, M. Hu, J. P. Strachan and H. Li, "Rescuing memristor-based neuromorphic design with high defects," 2017 54th ACM/EDAC/IEEE Design Automation Conference (DAC), Austin, TX, USA, 2017, pp. 1-6, doi: 10.1145/3061639.3062310.
- M. Hassan, C. Yang, C. Liu, H. H. Li and Y. Chen, "Hybrid spiking-based multi-layered self-learning neuromorphic system based on memristor crossbar arrays," Design, Automation & Test in Europe Conference & Exhibition (DATE), 2017, Lausanne, Switzerland, 2017, pp. 776-781, doi: 10.23919/DATE.2017.7927094.
- Liu, Q. Yang, C. Zhang, H. Jiang, Q. Wu and H. H. Li, "A memristor-based neuromorphic engine with a current sensing scheme for artificial neural network applications," 2017 22nd Asia and South Pacific Design Automation Conference (ASP-DAC), Chiba, Japan, 2017, pp. 647-652, doi: 10.1109/ASPDAC.2017.7858397.
- Liu et al., "A spiking neuromorphic design with resistive crossbar," 2015 52nd ACM/EDAC/IEEE Design Automation Conference (DAC), San Francisco, CA, USA, 2015, pp. 1-6, doi: 10.1145/2744769.2744783.