Elizabeth Qian
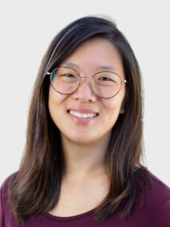
Fellowship
Hans Fischer Fellowship
Appointment
2023
Institution
Georgia Institute of Technology
Department
Daniel Guggenheim School of Aerospace Engineering
Hosts
Prof. Daniel Straub, Dr. Iason Papaioannou, Prof. Elisabeth Ullmann
Focus Group
Model Reduction for Structural Reliability Analysis
Short CV
Elizabeth Qian is a tenure-track Assistant Professor at the Georgia Institute of Technology, jointly appointed in the Schools of Aerospace Engineering and Computational Science and Engineering. Previously, she held the von Kármán Instructorship in applied and computational mathematics at the California Institute of Technology. She earned her bachelor’s and master’s degrees in aerospace engineering and her PhD in computational science and engineering from the Massachusetts Institute of Technology. Dr. Qian’s cross-disciplinary research develops new computational methods to enable and accelerate engineering decision-making, with particular focus on methods for scientific machine learning and projection-based model reduction, and multifidelity methods for optimization and uncertainty quantification. Dr. Qian’s research has been used in diverse applications across aerospace sciences including simulation of rocket combustors and uncertainty quantification for space mission design, and the methods are more broadly applicable across disciplines. Dr. Qian serves on the technical committees for Multidisciplinary Design Optimization and Non-deterministic Approaches in the American Institute of Aeronautics and Astronautics and is a member of the Society for Industrial and Applied Mathematics.
Selected Awards
- 2023, Hans Fischer Fellowship at the Institute for Advanced Study, Technical University of Munich
- 2023, one of 82 early career engineers selected nationwide to participate in the National Academy of Engineering’s Frontiers of Engineering Symposium
- 2022, World Congress on Computational Mechanics Best Presentation Award, postdoctoral category, International Association for Computational Mechanics Female Researchers Chapter
- 2022, Associated Students of Caltech (ASCIT) Teaching Award
- 2022, New Horizons Diversity, Equity, and Inclusion Award, Division of Engineering and Applied Sciences, Caltech
- 2020, SIAM Student Paper Prize
- 2014, Fannie and John Hertz Foundation Fellowship
- 2014, National Science Foundation Graduate Research Fellowship
- 2014, Fulbright Student Grant, Germany
Research Interests
Scientific machine learning, model reduction, multifidelity methods, optimization, uncertainty quantification, reliability analysis, inverse problems, data assimilation
Selected Publications
- Q. Wang, S. Gomez, P. Blonigan, A. Gregory, and E. Qian. P20. Physics of Fluids, 25(11):110818, 2013
- E. Qian, M. Grepl, K. Veroy, and K. Willcox. A certified trust region reduced basis approach to PDE-constrained optimization. SIAM Journal on Scientific Computing, 39(5):5–S460, 2017
- E. Qian, B. Peherstorfer, D. O’Malley, V. Vesselinov, and K. Willcox. Multifidelity Monte Carlo estimation of variance and sensitivity indices. SIAM/ASA Journal on Uncertainty Quantification, 6(2):683–706, 2018. Awarded a SIAM Student Paper Prize in 2020
- E. Qian, B. Kramer, B. Peherstorfer, and K. Willcox. Lift & Learn: Physics-informed machine learning for large-scale nonlinear dynamical systems. Physica D: Nonlinear Phenomena, 406:132401, 2020
- E. Qian, J. M. Tabeart, C. A. Beattie, S. Gugercin, J. Jiang, P. R. Kramer, and A. Narayan. Model reduction of linear dynamical systems via balancing for Bayesian inference. Journal of Scientific Computing, 91(29), 2022
- E. Qian, I.-G. Farcas, and K. Willcox. Reduced operator inference for nonlinear partial differential equations. SIAM Journal on Scientific Computing, 44(4):A1934–A1959, 2022
- G. Cataldo, E. Qian, and J. Auclair. Multifidelity uncertainty quantification and model validation of large-scale multidisciplinary systems. Journal of Astronomical Telescopes, Instruments, and Systems, 8(3):038001, 2022
- M. de Hoop, D. Z. Huang, E. Qian, and A. Stuart. The cost-accuracy trade-off in operator learning with neural networks. Journal of Machine Learning, 1(3):299–341, 2022