Heather J. Kulik
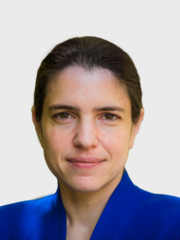
Fellowship
Hans Fischer Senior Fellowship
Appointment
2023
Institution
Massachusetts Institute of Technology
Department
Department of Chemical Engineering
Hosts:
Prof. Christopher J. Stein & Prof. Jennifer L.M. Rupp
Focus Group
Systematically Improvable Modeling of Electrochemical Processes
Short CV
Professor Heather J. Kulik is a tenured Associate Professor in the Departments of Chemical Engineering and Chemistry at MIT. She received her B.E. in Chemical Engineering from the Cooper Union in 2004 and her Ph.D. from the Department of Materials Science and Engineering at MIT in 2009. She completed postdoctoral training at Lawrence Livermore and Stanford, prior to joining MIT as a faculty member in November 2013, where she was promoted to associate without tenure (2019), with tenure (2021), and jointly appointed faculty in the Department of Chemistry (2022). Since starting her faculty position at MIT, she has authored or co-authored more than 140 peer reviewed scientific publications and presented over 200 invited national and international talks. Heather's research activities focus on developing computational workflows and machine learning models combined with first-principles and atomistic modeling for the discovery of new materials and catalysts as well as to develop mechanistic understanding in enzymes and non-biological catalysts.
Selected Awards
- 2023: AIChE CoMSEF Impact Award
- 2022: Distinguished Romberg Guest Professorship, University of Heidelberg
- 2021: Alfred P. Sloan Research Fellowship in Chemistry
- 2021: Molecular Systems Design & Engineering (2020) Outstanding Early-Career Paper Award
- 2020: DARPA Director’s Fellowship
- 2019: The Journal of Physical Chemistry B Lectureship (ACS PHYS Division)
- 2019: National Science Foundation CAREER Award
- 2019: AAAS Marion Milligan Mason Award
- 2018: Office of Naval Research Young Investigator Award
- 2018: ACS OpenEye Outstanding Junior Faculty Award in Computational Chemistry (ACS COMP Division)
Research Interests
Electronic structure, density functional theory, machine learning, catalysis, functional materials, enzymes, transition metal chemistry
Selected Publications
- Janet, J. P.; Ramesh, S.; Duan, C.; Kulik, H. J., Accurate Multiobjective Design in a Space of Millions of Transition Metal Complexes with Neural-Network-Driven Efficient Global Optimization. ACS Central Science, 6, 513-524 (2020).
- Nandy, A.; Duan, C.; Kulik, H. J., Using Machine Learning and Data Mining to Leverage Community Knowledge for the Engineering of Stable Metal-Organic Frameworks. Journal of the American Chemical Society, 143, 17535–17547 (2021).
- Nandy, A.; Duan, C.; Taylor, M. G.; Liu, F.; Steeves, A. H.; Kulik, H. J., Computational Discovery of Transition-Metal Complexes: From High-throughput Screening to Machine Learning. Chemical reviews, 121, 9927-10000 (2021).
- Duan, C.; Nandy, A.; Meyer, R.; Arunachalam, N.; Kulik, H. J., A Transferable Recommender Approach for Selecting the Best Density Functional Approximations in Chemical Discovery. Nature Computational Science, 3, 38-47 (2023).
- Duan, C.; Nandy, A.; Terrones, G.; Kastner, D. W.; Kulik, H. J., Active Learning Exploration of Transition Metal Complexes to Discover Method-Insensitive and Synthetically Accessible Chromophores. JACS Au, 3, 391-401 (2023).
- Nandy, A.; Yue, S.; Oh, C.; Duan, C.; Terrones, G. G.; Chung, Y. G.; Kulik, H. J., A Database of Ultrastable MOFs Reassembled from Stable Fragments with Machine Learning Models. Matter, 6, 1585-1603 (2023).
- Kastner, D. W.; Nandy, A.; Mehmood, R.; Kulik, H. J., Mechanistic Insights Into Substrate Positioning Across Non-heme Fe(II)/a-ketoglutarate-dependent Halogenases and Hydroxylases. ACS Catalysis, 13, 2489-2501 (2023).
- Adamji, H.; Nandy, A.; Kevlishvili, I.; Roman-Leshkov, Y.; Kulik, H. J., Computational Discovery of Stable Metal-Organic Frameworks for Methane-to-Methanol Catalysis. Journal of the American Chemical Society, 145, 14365-14378 (2023).
- Cytter, Y.; Nandy, A.; Duan, C.; Kulik, H. J., Insights into the deviation from piecewise linearity in transition metal complexes from supervised machine learning models. Physical Chemistry Chemical Physics, 25, 8103-8116 (2023).
- Cho, Y.; Nandy, A.; Duan, C.; Kulik, H. J., DFT-based Multireference Diagnostics in the Solid State: Application to Metal–organic Frameworks. Journal of Chemical Theory and Computation, 19, 190-197 (2023).